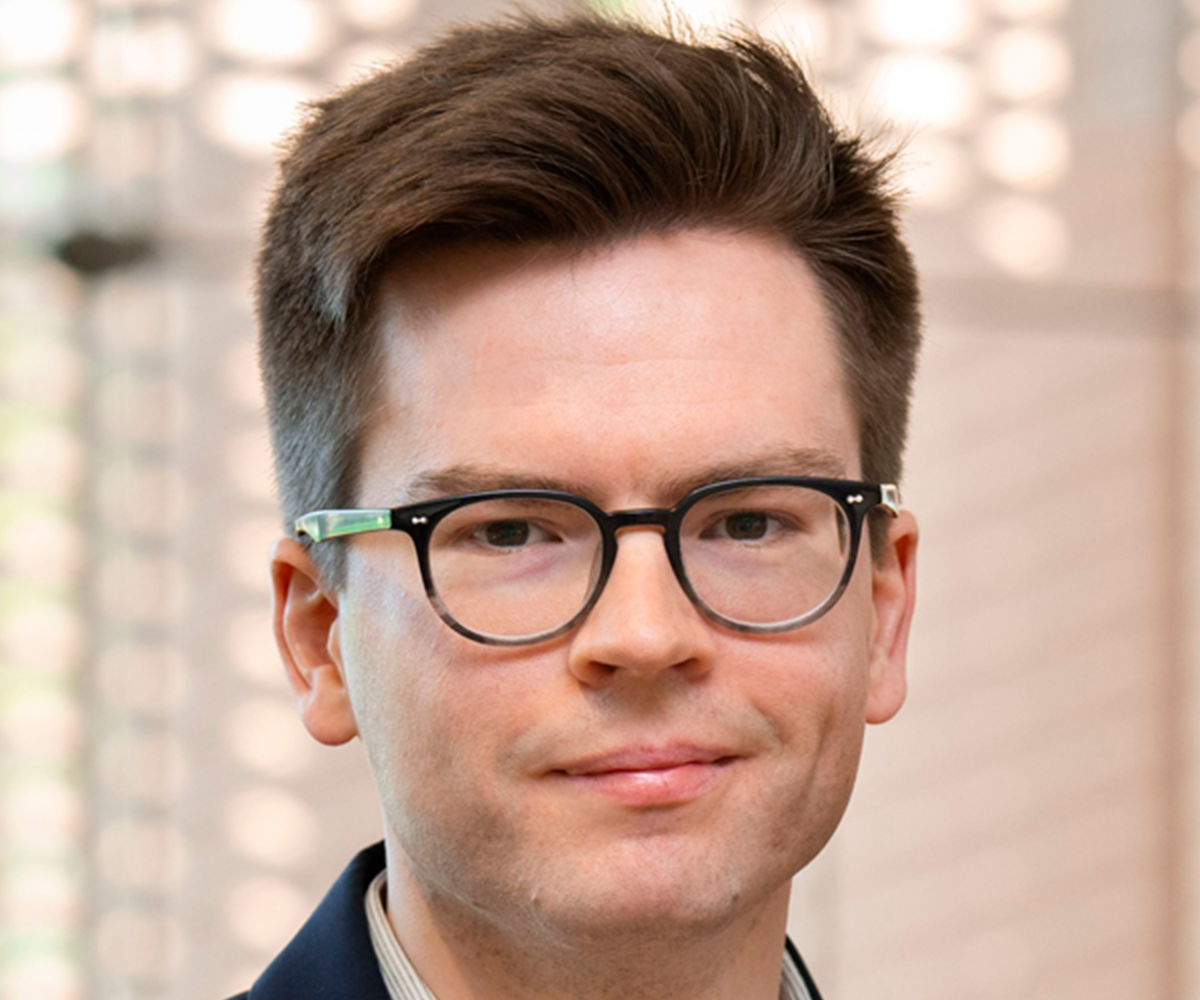
Generative models based on deep neural networks have emerged as a powerful framework for structure-based drug design by enabling more efficient exploration of chemical space, a universe estimated to contain some 1060 possible molecules. The most productive of those are trained on SMILES (for Simplified Molecular-Input Line-Entry System)—chemical language models that represent molecules as strings of text. Some fraction of SMILES strings generated by these models are not viable chemical structures, and researchers have strenuously sought to limit their generation or to correct for them. Ludwig Princeton’s Michael Skinnider showed in a Nature Machine Intelligence study solely authored by him that those efforts are unnecessary, and even detrimental: the ability to produce invalid outputs provides a self-corrective mechanism that filters low-likelihood samples from the language model output, improving the model’s performance. Further, enforcing valid outputs hampers learning and limits the exploration of chemical space. Invalid SMILES, he argues, are a feature of such models, not a bug. Beyond enabling more efficient drug design, optimized models were able to anticipate the existence of so far undiscovered dietary small molecules, which could provide a technological platform to identify diet-derived small molecules that modulate cancer progression or therapeutic efficacy.
Invalid SMILES are beneficial rather than detrimental to chemical language models
Nature Machine Intelligence, 2024 March 29