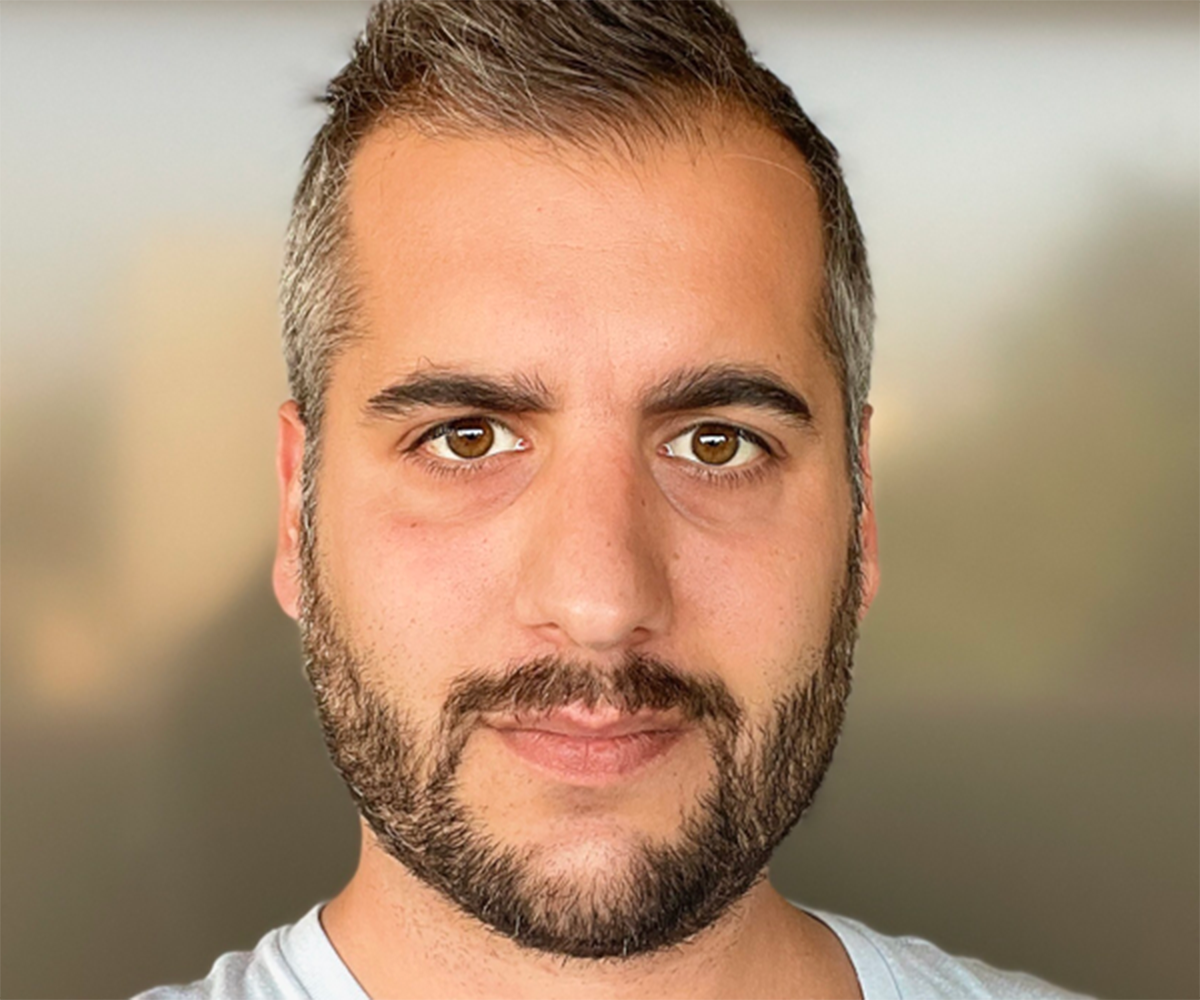
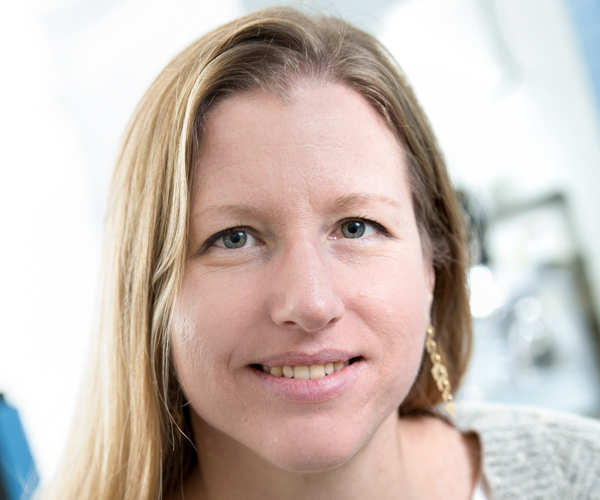
Researchers led by Ludwig Lausanne’s Florian Huber and Michal Bassani-Sternberg described in an October issue of Nature Biotechnology a start-to-finish computational pipeline for cancer vaccine design named NeoDisc. The pipeline integrates data from genomic analyses of the tumors and normal cells from the patient as well as transcriptomics and mass spectrometry-based immunopeptidomics to generate its output. Aside from neoantigens, NeoDisc detects erroneously expressed noncoding DNA, tumor-associated genes and other aberrantly expressed gene products in cancer cells as well as viral antigens in relevant cases. It then applies both machine learning and rule-based algorithms to prioritize those most likely to elicit T cell responses and designs a personalized cancer vaccine for a patient. NeoDisc also ranks the potentially targetable antigens it detects and analyzes cancer cell heterogeneity within tumors. Further, it can detect potential defects in the machinery of antigen presentation—flagging a key mechanism of immune evasion in tumors—and thus help select patients likely to benefit from personalized immunotherapies. Michal, Florian and colleagues demonstrated that NeoDisc provides a more accurate selection of effective cancer antigens for vaccines and adoptive cell therapies than do other computational tools currently used for that purpose. The computational pipeline at the heart of NeoDisc is already being used in clinical trials of personalized cancer vaccines and adoptive cell therapies in Lausanne.
A comprehensive proteogenomic pipeline for neoantigen discovery to advance personalized cancer immunotherapy
Nature Biotechnology, 2024 October 11