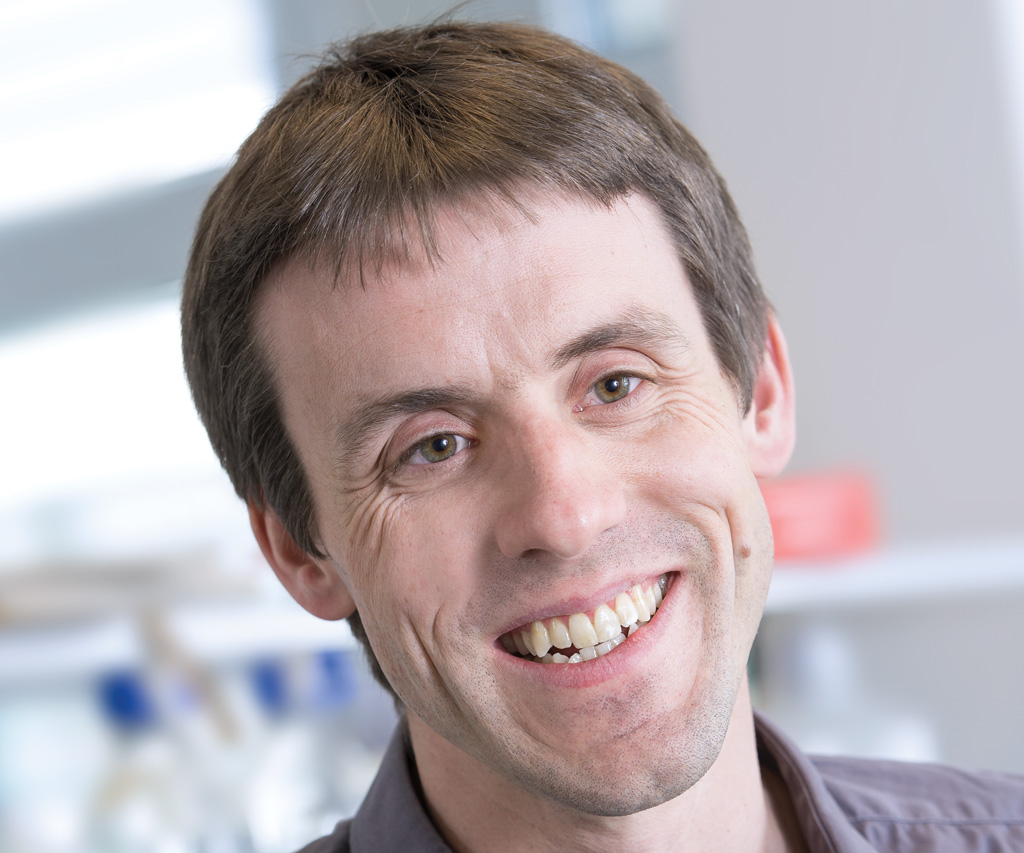
Cancer research is undergoing a transformative shift towards a data-driven science. The pivotal role of AI in this evolution is undeniable. In our research, we prioritize creating computational models that utilize AI’s predictive capabilities, while also ensuring they remain interpretable. This emphasis on interpretability aids in comprehending the rationale behind AI predictions.
DAVID GFELLER
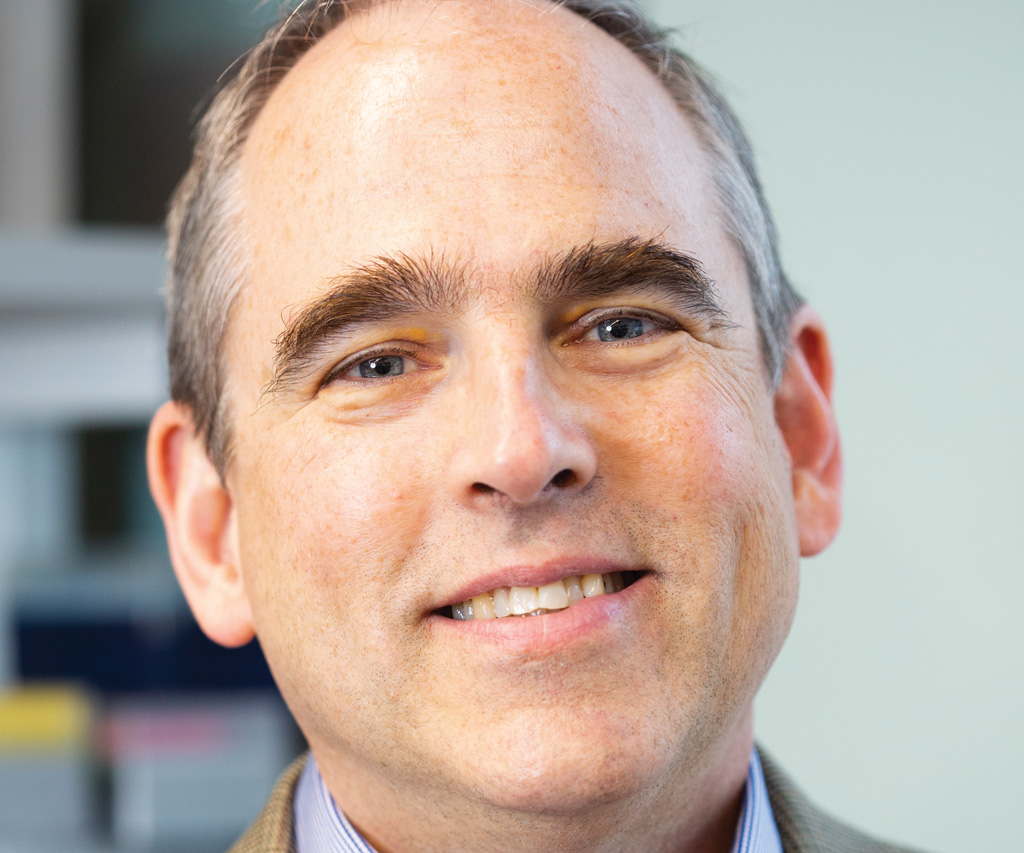
We use AI and machine learning (AI/ML) to study the tumor-immune microenvironment in highly multiplexed and traditional tissue images. AI/ML can be highly effective in this setting, revealing morphological and molecular features that are not always discernable to human experts. We are aiming for machine-human collaboration that uses AI to accelerate scientific discovery and improve diagnosis.
PETER SORGER
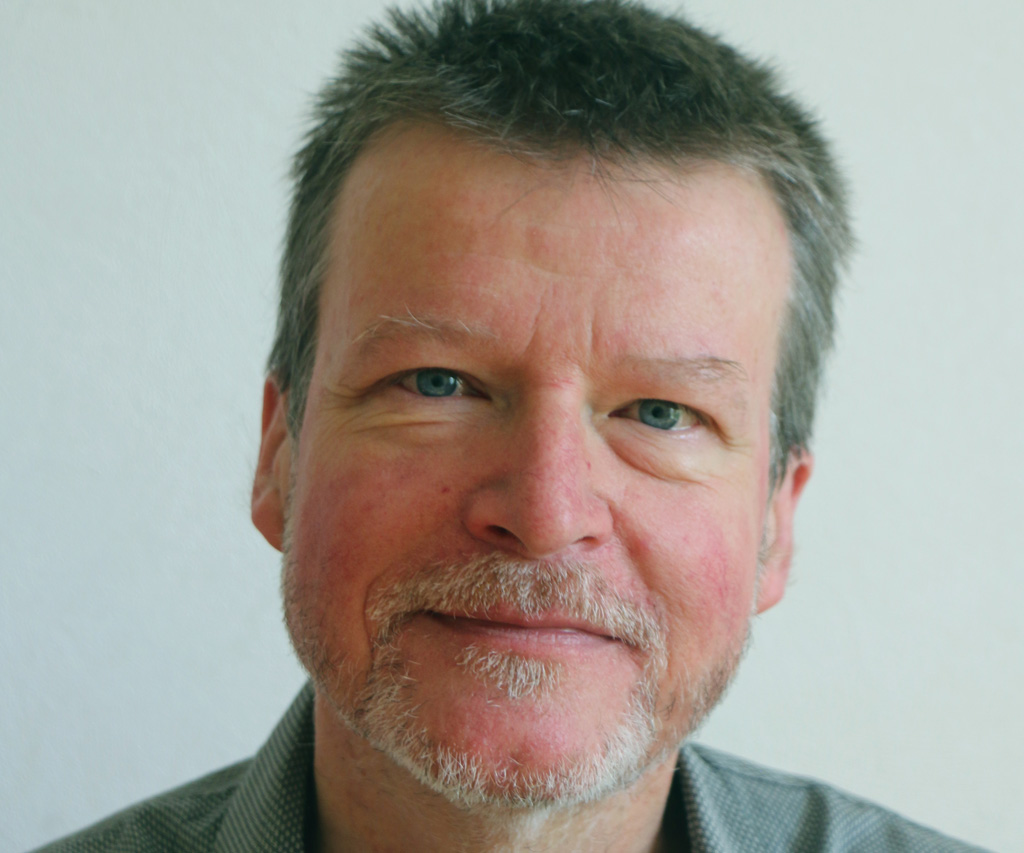
We showed that machine learning can improve the prioritization of HLA-I neoantigens as targets for cancer immunotherapy. These predictors are included in our NeoDisc neoantigen prediction pipeline and used in personalized cancer vaccine and T cell programs at the CHUV. Future patient data will allow us to improve and extend the predictors to HLA-II binding neoantigens.
MARKUS MÜLLER
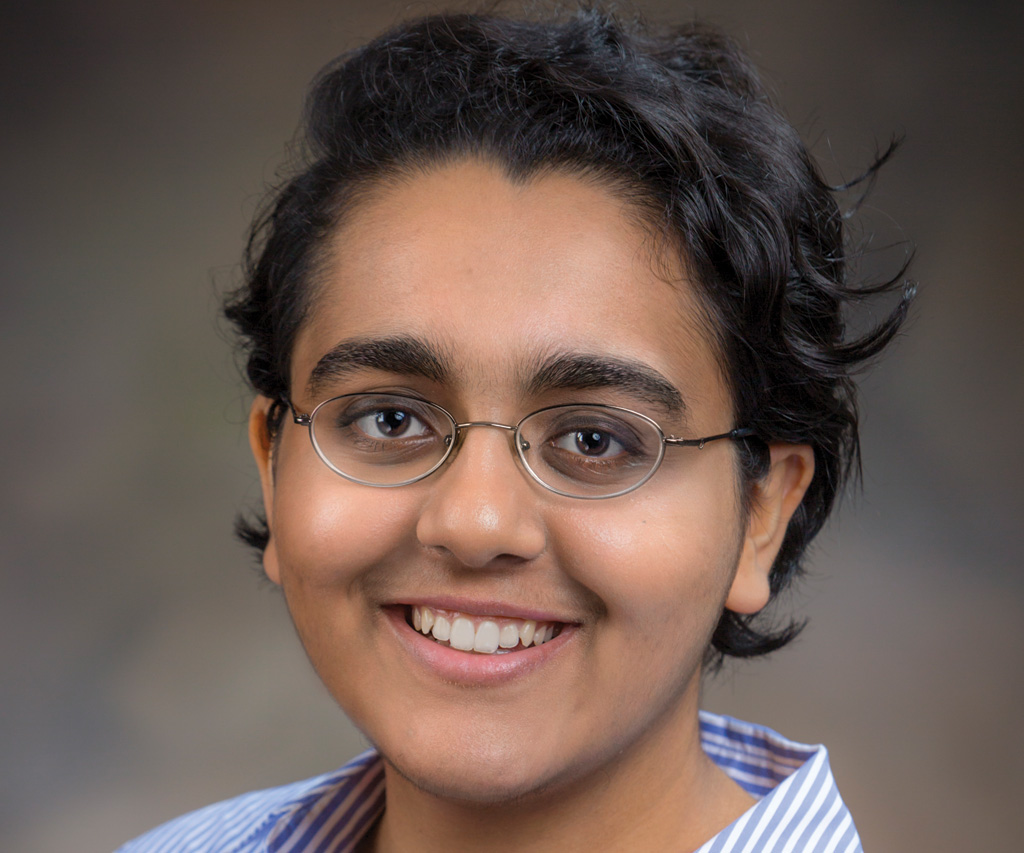
I don’t currently use AI in my bioinformatics projects. However, there are myriad applications for it in research and medicine. For example, AI can help clinicians diagnose cancers by analyzing imaging scans. It can also assist researchers to identify biomarkers of therapeutic response in large clinical trial datasets.
NEHA WALI
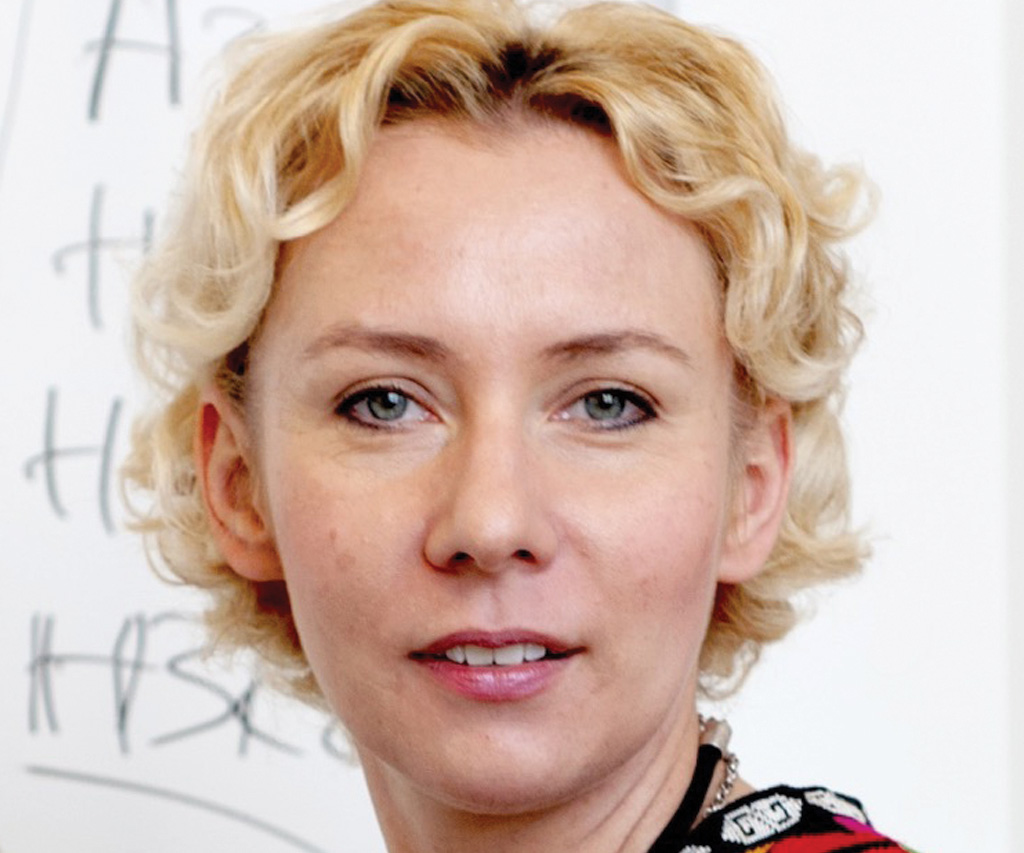
We are using AI to read the regulatory code of the genome – how transcription factors, the “master regulators” of cell type identity, activate regulatory elements throughout the genome, and how such regulatory elements modulate expression of their target genes.
JOANNA WYSOCKA
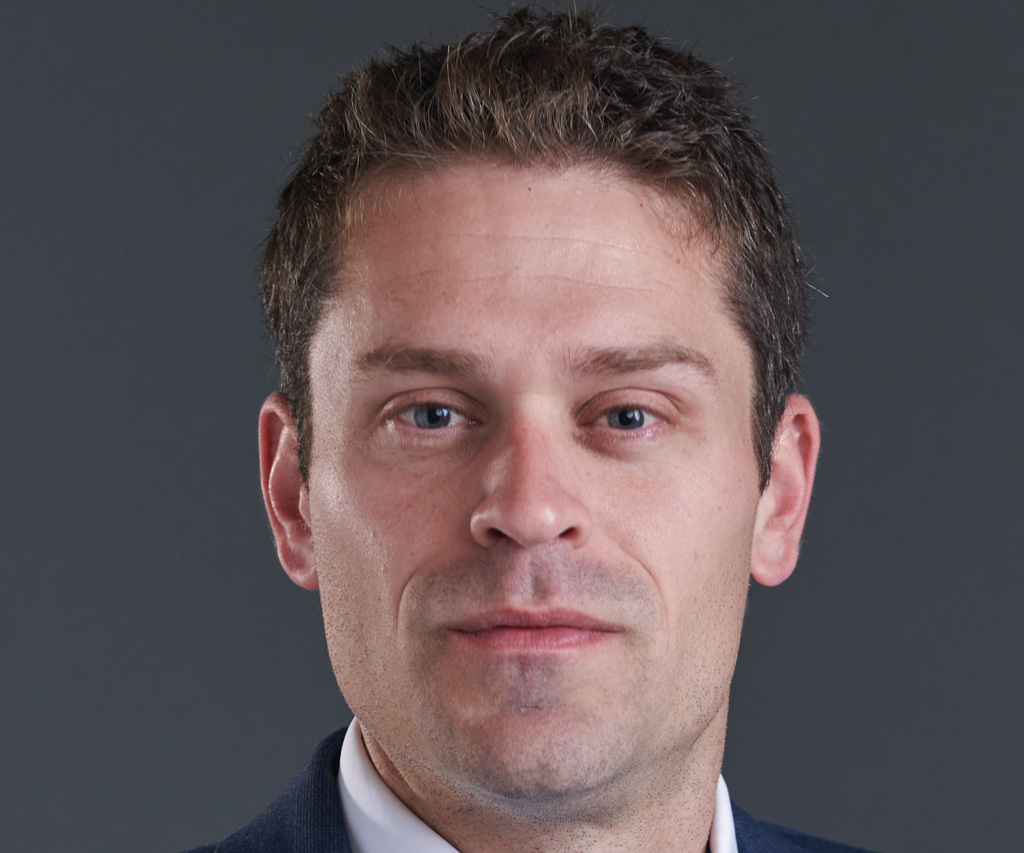
We find machine learning and large data sets being applied to a wide variety of problems in our group but we find it is critical to remember that these powerful tools are not magic and NOT a substitute for a sound hypothesis, a deep understanding of the data and a recognition of the limitations of the experimental data.
CHRISTOPHER DOUVILLE
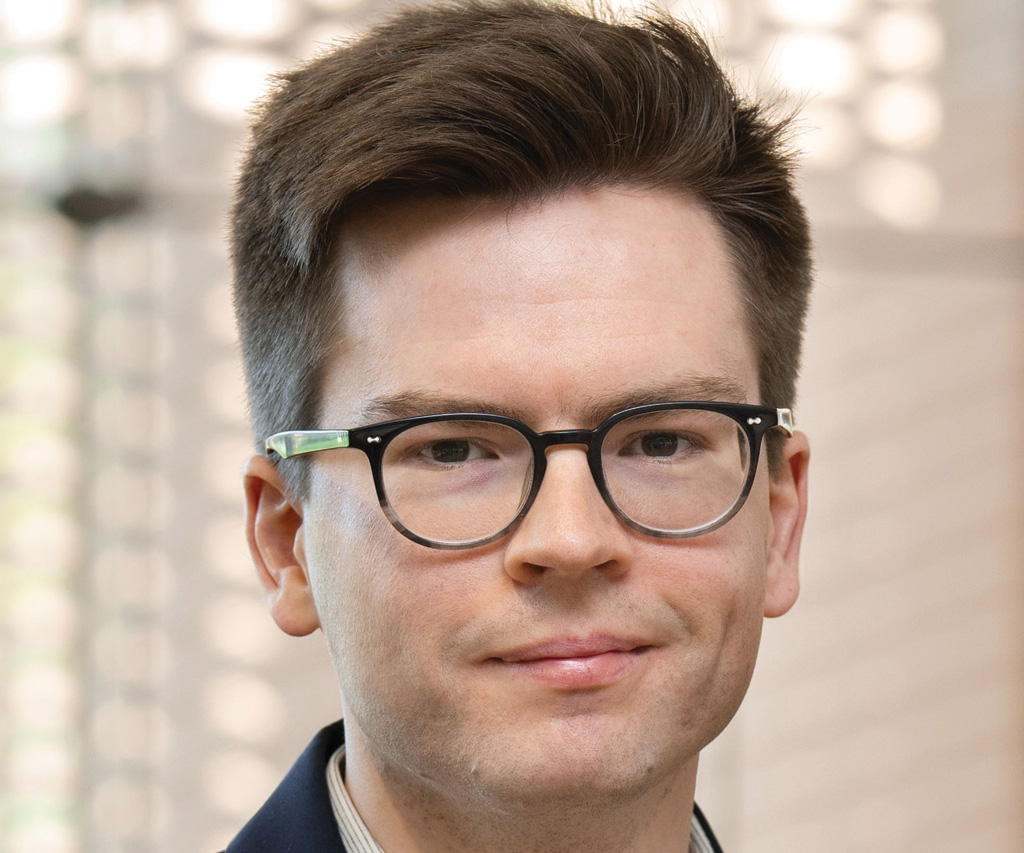
My lab is developing and applying chemical AI to mass spectrometry-based metabolomics data to discover new human and microbiome-derived metabolites. We are excited both about the possibility of using AI to extract more value from existing or routinely-collected datasets, and allowing us to collect even larger datasets through more complex and ambitious experiments with the expectation that we will be able to sift through huge amounts of data to ultimately arrive at new biological and clinical insights.